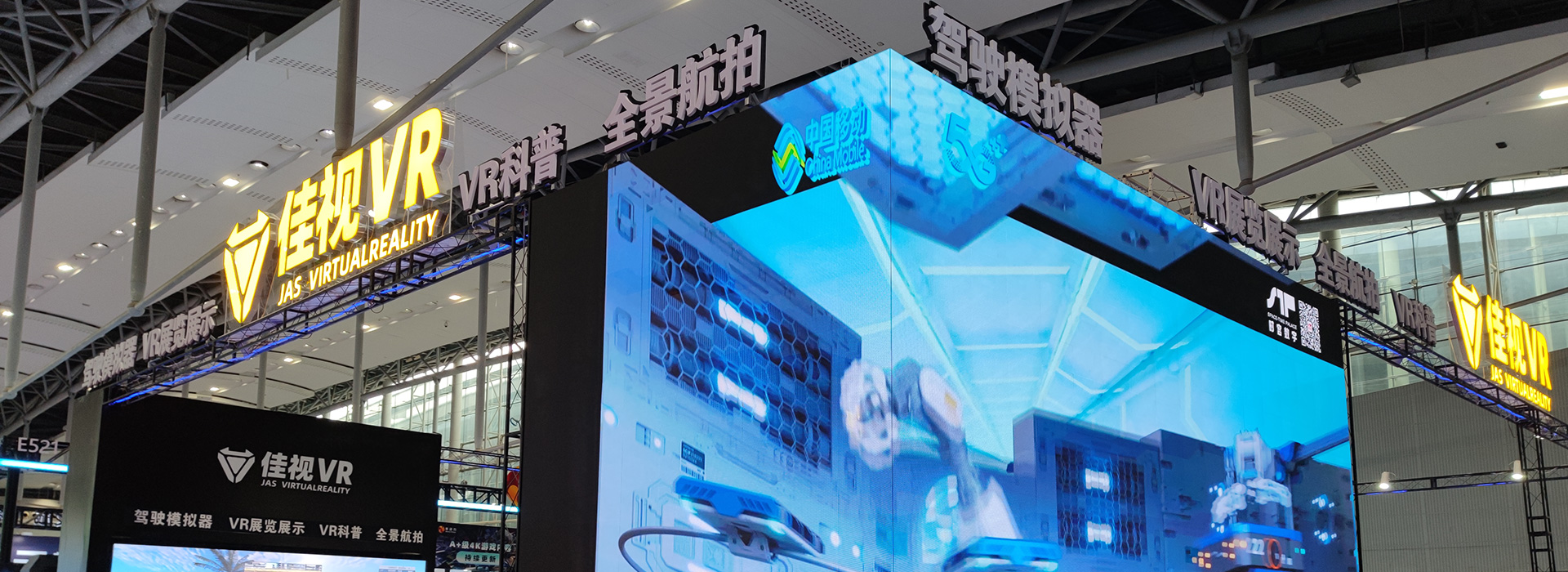
Core Applications and Cutting-Edge Exploration of Automotive Driving Simulators in Autonomous Driving Technology
With the rapid iteration of artificial intelligence and autonomous driving technologies, driverless vehicles have gradually moved from laboratory concepts to commercial implementation. In the process of technological development and validation, automotive driving simulators have become indispensable tools due to their advantages of high efficiency, safety, and low cost. This article will delve into how automotive driving simulators empower the research and testing of autonomous driving technology, covering technical principles, practical applications, industry case studies, and future development directions.
1. Core Technologies and Functions of Automotive Driving Simulators
An automotive driving simulator is a device that constructs virtual environments and simulates real-world driving scenarios using computer technology. Its core functions include vehicle dynamics modeling, environmental scene generation, sensor data simulation, and user interaction control. Below is a detailed analysis of the key technologies:
1.1 Vehicle Dynamics Modeling
The simulation system must accurately replicate the physical motion characteristics of a vehicle. By establishing a multi-body dynamics model, the simulator can calculate the impact of operations such as steering, acceleration, and braking on the vehicle’s trajectory. For example, the distribution of lateral and inertial forces during steering, as well as the dynamic response of the suspension system. High-precision modeling can reflect subtle differences in real-world driving, providing a reliable testing benchmark for autonomous driving algorithms.
1.2 Environmental Scene Generation
The diversity of virtual scenes directly affects simulation effectiveness. Using procedural generation technology, urban roads, highways, rural paths, and other scenarios can be quickly generated, with support for dynamic weather changes, traffic flow density adjustments, and the insertion of sudden obstacles. For instance, simulating slippery roads during heavy rain or emergency situations like pedestrians suddenly crossing the street.
1.3 Sensor Data Simulation
Autonomous driving relies on multi-sensor fusion perception, including LiDAR, cameras, and millimeter-wave radar. The simulator must generate digital signals consistent with real sensors, such as point clouds, image frames, and distance detection data. For example, in a virtual environment, cameras can “see” traffic signs, lane markings, and obstacles identical to those in the real world.
1.4 Human-Machine Interaction and Driving Behavior Modeling
Some simulators support customizable driver behavior models to test how autonomous vehicles adapt to human driving behaviors. For example, machine learning algorithms can generate “virtual human drivers” with different driving styles to test decision-making optimization in complex road conditions.
2. Key Application Scenarios in Autonomous Driving Technology
Driving simulators play a crucial role throughout the lifecycle of autonomous driving technology development, from algorithm validation to system integration.
2.1 Decision Algorithm Optimization
Challenge: Autonomous vehicles must process traffic rules, road conditions, and emergencies in real time, with the core being path planning and behavioral decision-making algorithms.
Solutions:
- Multi-scenario testing: The simulator can quickly switch traffic light modes (e.g., sudden yellow lights), pedestrian behaviors (e.g., sudden crossings), and vehicle dynamics (e.g., emergency braking) to test the robustness of decision algorithms.
- Data-driven improvements: By collecting failure cases (e.g., collisions) in the simulator, deep learning model parameters can be optimized. For example, Waymo used simulation tests to correct approximately 20% of emergency avoidance strategies.
2.2 Sensor Fusion and Calibration
Challenge: Differences in sensor accuracy, latency, and field of view require fusion algorithms to integrate environmental information.
Solutions:
- Sensor failure testing: Simulate scenarios like radar malfunctions or camera obstructions to verify the reliability of redundant systems.
- Parameter calibration: Precisely control the distance and angle of target objects in virtual environments to ensure sensor data aligns with the physical world. For instance, Baidu Apollo calibrated camera parameters by simulating day-night lighting changes.
2.3 Safety Verification and Fault Testing
Challenge: Autonomous driving systems must meet high safety standards (e.g., accident-free performance over millions of kilometers).
Solutions:
- Extreme condition testing: The simulator allows repeated testing of extreme scenarios (e.g., icy roads or erratic pedestrians) in a short time, reducing real-world testing costs.
- Fault injection: Introduce system vulnerabilities (e.g., positioning module failures) to evaluate the effectiveness of emergency mechanisms. For example, Mobileye’s Responsibility-Sensitive Safety (RSS) model validated collision prevention capabilities through simulation.
2.4 Driver Intervention and Collaboration Modes
Challenge: For Level 3 and above autonomous systems, the interaction logic between drivers and vehicles must be meticulously designed.
Solutions:
- Human-machine co-driving scenarios: The simulator can simulate “takeover failures” or “misoperations” to optimize human-machine interface (HMI) design. For instance, Tesla’s FSD Beta improved alert prompts by simulating emergency takeover scenarios.
- Remote monitoring testing: Future autonomous taxis (Robotaxis) require testing the response speed of cloud-based control systems, and simulators can replicate network latency or packet loss issues.
3. Industry Practices and Case Studies
Below are key examples of how automakers and tech companies integrate driving simulators into autonomous driving R&D:
3.1 Tesla: Virtual World and Real-World Data Loop
Tesla uses its “Shadow Mode” combined with simulators to achieve data-driven iteration. Vehicle sensors collect real-time scene data, upload it to the cloud, and filter potential corner cases to generate high-fidelity simulation environments. The simulator and real-world testing form a closed loop, continuously optimizing the Autopilot algorithm. According to public data, Tesla’s simulated test mileage has long exceeded billions of kilometers.
3.2 Waymo: Carcraft Virtual City Network
Waymo, a subsidiary of Google, launched the Carcraft platform, which includes a simulation database with millions of virtual scenarios. Features of the platform include:
- Dynamic traffic flow simulation: Simulates real-world urban traffic patterns (e.g., rush hour congestion) based on historical data.
- Automated testing pipeline: Uses reinforcement learning to automatically generate extreme cases and validate solutions. Over 99.9% of Waymo’s testing mileage comes from simulations.
3.3 Baidu Apollo: Open-Source Simulation Platform Apollo SIME
Apollo SIME provides high-definition map editing, large-scale parallel scenario simulation, and multi-sensor joint simulation. Its open ecosystem attracts developers to contribute test cases, covering road networks in over 200 Chinese cities. For example, Baidu collaborated with the Baoding government to import real traffic flow data into the simulation platform, accelerating Robotaxi commercialization.
4. Current Limitations and Challenges
Despite their widespread use, simulators still face the following technical bottlenecks:
- Insufficient physical realism: Current simulation software has limited accuracy in replicating extreme physical conditions (e.g., high-speed collisions) and requires more advanced physics engines.
- Data privacy and security: Large amounts of real-world test data used in simulations without anonymization pose privacy risks.
- High computational costs: High-precision simulations require powerful GPU clusters, which are unaffordable for small and medium-sized teams.
5. Future Trends and Development Directions
To overcome current limitations, simulation technology is evolving in the following directions:
- Digital Twin integration: Combines IoT technology to achieve real-time synchronization between physical vehicles and virtual models, improving testing efficiency.
- Metaverse platform fusion: Leverages Web3.0 and cloud computing to build an open simulation ecosystem shared by global developers.
- Generative AI-assisted testing: Uses large models like GPT to automatically generate complex scenario scripts, such as “emergency lane changes during a multi-vehicle race in heavy rain.”