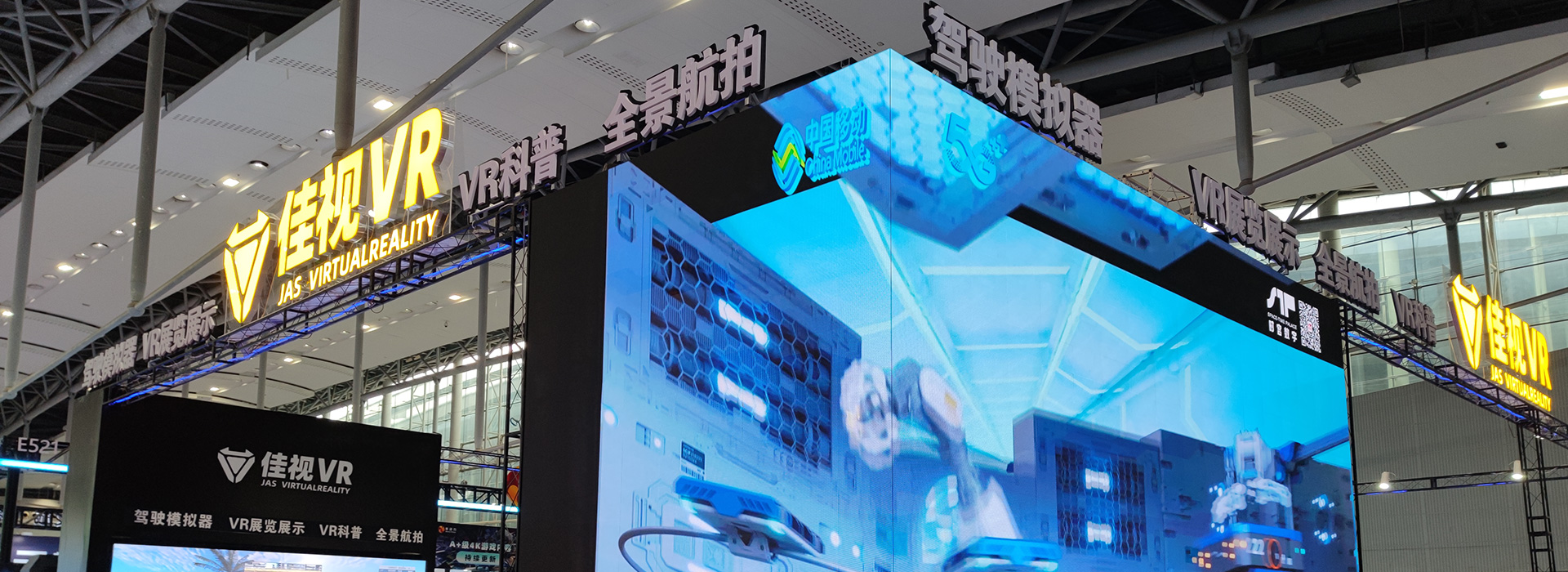
The Pivotal Role of 5G-Powered Automotive Driving Simulators in Autonomous Vehicle Development
With the commercialization of fifth-generation mobile networks (5G) accelerating globally, the automotive industry is undergoing an unprecedented intelligent revolution. 5G’s ultra-high bandwidth, ultra-low latency, and massive connectivity are providing critical infrastructure support for autonomous driving technologies. In this context, automotive driving simulators—as essential tools for AV R&D, testing, and validation—are being fundamentally redefined and elevated in value.
Statistics indicate the global autonomous vehicle market will reach $60 billion by 2025, growing at a 36% CAGR. 5G deployment is significantly accelerating AV commercialization timelines. Within this process, driving simulators have evolved from traditional driver training tools into multifunctional platforms for AV algorithm development, system verification, and human-machine interaction research. Particularly in 5G environments, simulators can construct highly realistic virtual testing scenarios, dramatically reducing real-world testing costs and risks while improving development efficiency.
This article comprehensively examines the critical role of 5G-enabled driving simulators in autonomous driving from technical principles, application scenarios, advantages, and future trends, while analyzing their profound impact on the automotive ecosystem.
2. Technological Evolution of Driving Simulators Enabled by 5G
2.1 Developmental Milestones
Since their inception in the mid-20th century, driving simulators have progressed through four generations:
- 1st Gen (1950s-60s): Electro-mechanical systems with basic projection for fundamental skill training.
- 2nd Gen (1970s-80s): Incorporated computer graphics for visual simulation.
- 3rd Gen (1990s-2000s): Achieved 3D real-time rendering and limited physical feedback.
- 4th Gen (Present): Integrates high-fidelity graphics, multi-DOF motion platforms, force feedback, and VR for near-realistic experiences.
2.2 5G’s Transformative Enhancements
5G brings qualitative leaps:
- Ultra-Low Latency (1ms): Enables real-time interaction between simulators, cloud computing, and infrastructure, ensuring seamless algorithm testing.
- Multi-Access Edge Computing (MEC): Offloads compute-intensive tasks (e.g., traffic flow simulations) to network edges.
- Massive Connectivity (1M devices/km²): Supports complex scenarios with numerous agents (vehicles, pedestrians, IoT devices) for comprehensive cooperative driving tests.
- Network Slicing: Guarantees QoS for bandwidth-sensitive real-time simulations.
2.3 System Architecture
A “Cloud-Edge-Device” framework:
- Device Layer: High-fidelity simulator cabins with VR headsets, force-feedback systems, and virtual sensor models.
- Edge Layer: MEC nodes handle real-time physics engines and local traffic simulations.
- Cloud Layer: Provides large-scale scenario storage, HD maps, and weather modeling.
- 5G Network: Acts as the nervous system, with network slicing prioritizing critical tasks.
3. Core Applications in Autonomous Driving
3.1 Algorithm Development & Validation
- Perception Training: Generates diverse sensor data (cameras, LiDAR) under varied conditions (weather, lighting) for object detection and sensor fusion algorithms.
- Decision-Making Tests: Simulates edge cases (e.g., jaywalking pedestrians) via 5G-connected large-scale traffic models.
- Control Verification: High-precision vehicle dynamics models run at the edge, enabling safe testing of emergency maneuvers.
3.2 Extreme & Hazardous Scenario Testing
- Rare Events: Child darting into traffic, falling cargo—reproduced reliably.
- Adverse Conditions: Flooded roads, snow-covered lanes, and system failures (e.g., sensor outages).
3.3 V2X & Cooperative Driving
- Large-Scale V2X Simulations: Hundreds of connected vehicles and smart traffic lights interact in virtual cities.
- Network Heterogeneity Testing: Validates AV performance under unstable 5G coverage.
3.4 Human-Machine Interaction
- Takeover Studies: Evaluates driver responsiveness during transitions from autonomous to manual mode.
- Shared Control: Tests arbitration mechanisms for human-AV control conflicts.
4. Advantages Over Traditional Methods
- 100–1000x Cost Reduction: Eliminates real-vehicle depreciation, fuel, and crash costs.
- Exponential Efficiency: Millions of virtual kilometers tested daily via parallel cloud simulations.
- Systematic Coverage: Replicates rare scenario combinations (e.g., glare + wet roads + sudden braking).
- Data-Driven Development: Enables closed-loop learning and digital twin comparisons.
5. Challenges & Solutions
- Fidelity vs. Real-Time Balance: Tiered physics modeling and dynamic rendering optimization.
- Distributed Computing for Large Scenarios: Spatial partitioning and edge-based load balancing.
- Sensor Realism: Physical sensor modeling with noise injection.
- Security: End-to-end encryption and blockchain-based access control.
6. Future Trends
- Digital Twin Integration: Real-time synchronization with smart city data.
- AI-Generated Scenarios: GANs create adversarial edge cases; reinforcement learning environments.
- Simulation-as-a-Service: Cloud-based testing platforms and scenario marketplaces.
- Standardization: Regulatory acceptance of virtual testing for AV certification.
7. Conclusion: The Virtual Foundation for Autonomous Futures
5G-powered simulators are no longer mere training tools but have become indispensable platforms for AV development. By enabling safe, scalable, and cost-effective validation, they address the critical bottleneck of testing billions of driving miles. As 5G, AI, and digital twin technologies mature, these simulators will further blur the lines between virtual and real worlds, reshaping automotive R&D paradigms and accelerating the advent of safe, sustainable autonomous transportation.